Contrast sensitivity functions in autoencoders

Differences and parallels between human vision and the vision of artificial neural networks.
The computer vision process can be subdivided into six main areas:
- Low level vision
- Sensing: It is the process that leads us to obtain a visual image
- Preprocessing: It deals with noise reduction techniques and image detail enrichment
- Segmentation: It is the process that partitions an image into objects of interest.
- Low level vision
- Description: Deals with the computation of useful features to differentiate one type of object from another.
- Recognition: It is the process that identifies those objects.
Within these mathematical models that can act and work simulating human vision are the so-called convolutional neural networks where artificial neurons correspond to receptive fields in a very similar way to the neurons in the primary visual cortex of a biological brain. These networks are made up of many process layers, very effective for artificial vision tasks, such as image classification and segmentation, among other applications.
However, recent research carried out by an international team made up of researchers from the Image Processing Laboratory of the University of Valencia, the Computer Vision Center of the Autonomous University of Barcelona and the CSIC Institute of Optics has carried out a comparative study of various neural networks reached the following conclusions:
Researchers have trained a very popular type of simple convolutional neural network, with few layers, to do basic image restoration operations (such as denoising or contrast enhancement) that are known to be done in the human visual system: in that In this case, the neural network also develops other properties similar to those of human vision, such as similar contrast sensitivity curves.
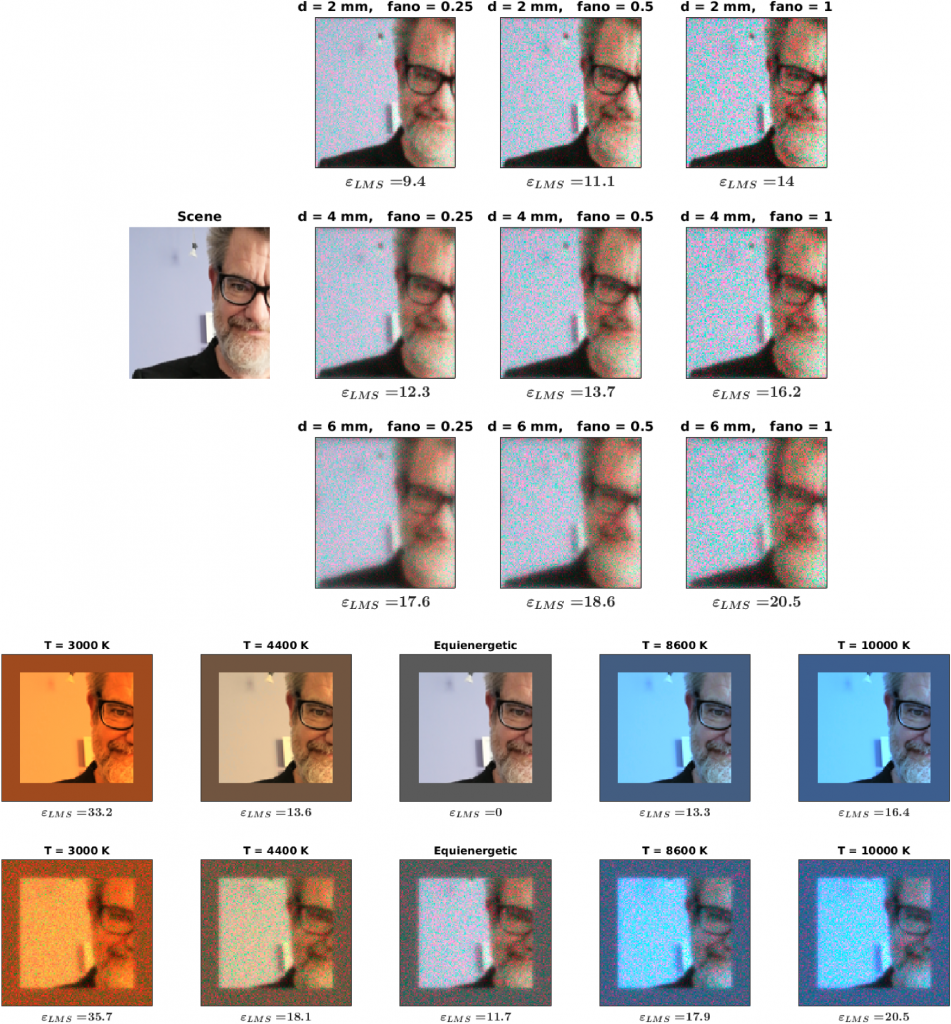
functional objectives. Possible low-level goals of autoencoders are to compensate for the following distortions in visual input.
What is the contrast sensitivity feature?
The Contrast Sensitivity Function (CSF) is a parameter that helps us to evaluate the quality of the visual system, since it provides us with subjective information on how people see the shapes of an object, detecting the presence of minimal differences in luminosity between objects or areas.
Following on from this result, the researchers have shown that the deepest convolutional neural networks (which have demonstrated their ability to solve complex image classification problems using millions of parameters,
learning with large databases and capable, for example, of recognizing emotions in images of human faces), trained for the same image restoration task, perform it better than simple networks but emulate other properties of human vision (such as contrast sensitivity functions mentioned above).
This interesting low-level result (for the explored networks) is not necessarily in contradiction to other work showing advances of deeper networks in modeling higher-level vision targets. However, they do provide a warning about caution in the use of convolutional neural networks in vision science, because the use of simplified units or unrealistic architectures in goal optimization can lead to models of effectiveness. limited or that make it difficult to understand human vision.
Related news
Two photographs of María Egües Ortiz (1917-2008): a periscopic gaze in times of misty silence
Madrid / July 26, 2023This essay published by our colleague Sergio Barbero from the IVIS group focuses on the figure of María Egües Ortiz (...
Presentation of the Exhibition: “The beginnings of the Institute of Optics ‘Daza de Valdés’
Madrid / November 18, 2022On the occasion of the 75th anniversary of the creation of the “Daza de Valdés” Institute of Optics we inaugurate an...
Spectral Pre-Adaptation for Restoring Real-World Blurred Images using Standard Deconvolution Methods
Do you want to see a demo of how the best image restoration algorithms work?Classic image blur correction models are based on assumptions that are...